Data science has rapidly transformed the way we understand and interact with the world. What started as a field rooted in statistics and computer science has evolved into a critical driver of innovation across industries. Data science has proven to be a transformative force, and by understanding its evolution and looking toward its future, we gain valuable insight into how it will continue to shape the modern world.
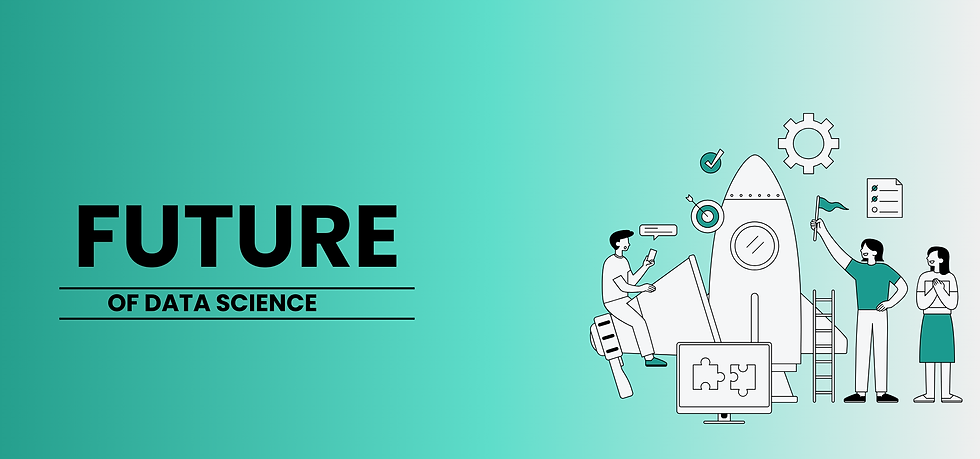
The Early Stages of Data Science
Data science traces its roots to traditional statistics, which has been used for centuries to analyze data. In the early 20th century, statistical methods like regression analysis and probability theory played key roles in interpreting data. This period focused on data collection, analysis, and interpretation within specific domains such as economics, psychology, and medicine.
In the 1960s and 1970s, advances in computing power enabled more complex calculations to be performed quickly. The rise of computers created a need for data storage and retrieval systems, marking the early stages of data management. During this time, data analysis was primarily manual, and data scientists were usually statisticians or mathematicians using a limited set of tools to interpret data.
The Rise of Big Data and Machine Learning
The real leap in data science came in the 2000s with the rise of "big data." The proliferation of the internet, social media, sensors, and mobile devices led to an explosion of data, that traditional methods couldn't process. Organizations began to realize that the insights hidden in these vast amounts of data could offer significant advantages in decision-making, business strategy, and customer experience.
During this time, machine learning (ML) emerged as a key discipline within data science. ML, a subset of artificial intelligence (AI), enables computers to learn patterns from data without being explicitly programmed. This shift allowed data scientists to develop predictive models and algorithms that could automate decision-making and identify trends previously hidden in the data.
The development of algorithms such as decision trees, neural networks, and support vector machines (SVMs) opened new avenues for automated analysis. This transformation also led to the creation of platforms and tools for handling big data, such as Hadoop, Spark, and cloud computing services, which allowed data scientists to process data faster and more efficiently.
Data Science in Action: Industries and Applications
Today, data science drives many innovations across industries. In healthcare, for example, machine learning models predict patient outcomes, optimize treatment plans, and identify disease outbreaks. In finance, data science has revolutionized risk management, fraud detection, and algorithmic trading. E-commerce platforms like Amazon and Netflix rely heavily on data science to deliver personalized recommendations based on user behavior.
The field has also seen significant advances in natural language processing (NLP), which enables computers to understand, interpret, and generate human language. This technology powers everything from chatbots to virtual assistants like Siri and Alexa, improving customer interactions and enhancing business operations.
The Future of Data Science Innovation
Looking forward, the future of data science is filled with exciting possibilities. As the volume of data continues to grow, the demand for more advanced and scalable methods for processing and analyzing it will increase. Several key trends are expected to shape the future of data science:
AI and Automation: AI-powered tools are likely to continue evolving, making it easier for businesses and individuals to automate data analysis. With the advent of AutoML (automated machine learning), developing machine learning models will become more accessible, enabling non-experts to build powerful models with minimal coding knowledge.
Ethics and Bias in AI: As data science becomes more integrated into decision-making, the ethical implications of its use are gaining importance. Issues such as data privacy, algorithmic bias, and fairness will need to be addressed to ensure that AI and data science are used responsibly. Researchers are working on frameworks to make data science more transparent and accountable.
Quantum Computing: Quantum computing holds the potential to revolutionize data science by processing massive datasets at speeds far beyond current capabilities. This could unlock new possibilities for solving complex problems in fields like cryptography, drug discovery, and climate modeling.
Edge Computing: As IoT devices become more pervasive, edge computing is expected to gain traction. This involves processing data closer to where it is generated (at the "edge" of the network) rather than relying on centralized cloud servers. This will reduce latency and improve real-time decision-making in applications like autonomous vehicles and industrial monitoring.
Data-Driven Personalization: In the future, businesses will continue to use data science to deliver hyper-personalized experiences. By leveraging advanced machine learning models, companies will create highly individualized offerings, from personalized shopping experiences to tailored content recommendations.
Collaboration Between Humans and Machines: Rather than replacing human jobs, data science and AI will likely enhance human decision-making. By combining human intuition and creativity with the computational power of machines, organizations can make more informed decisions and drive innovation.
For those looking to be part of this future, exploring data science training classes in Noida, Delhi, Pune, and other cities across India can provide aspiring professionals with the skills needed to collaborate with machines and drive these innovations forward.
Conclusion
The evolution of data science has been nothing short of remarkable. From its origins in statistics to its present-day applications in artificial intelligence and machine learning, data science has become a cornerstone of innovation across industries. As technology continues to advance, the future of data science promises even more transformative changes, particularly with the integration of AI, quantum computing, and ethical frameworks.
Ultimately, the future of data science lies in its ability to drive smarter, data-informed decisions that can tackle some of the world’s most pressing challenges, from healthcare to climate change. As we move forward, data science will remain at the forefront of technological innovation, shaping the way we live, work, and solve problems.
Comentários